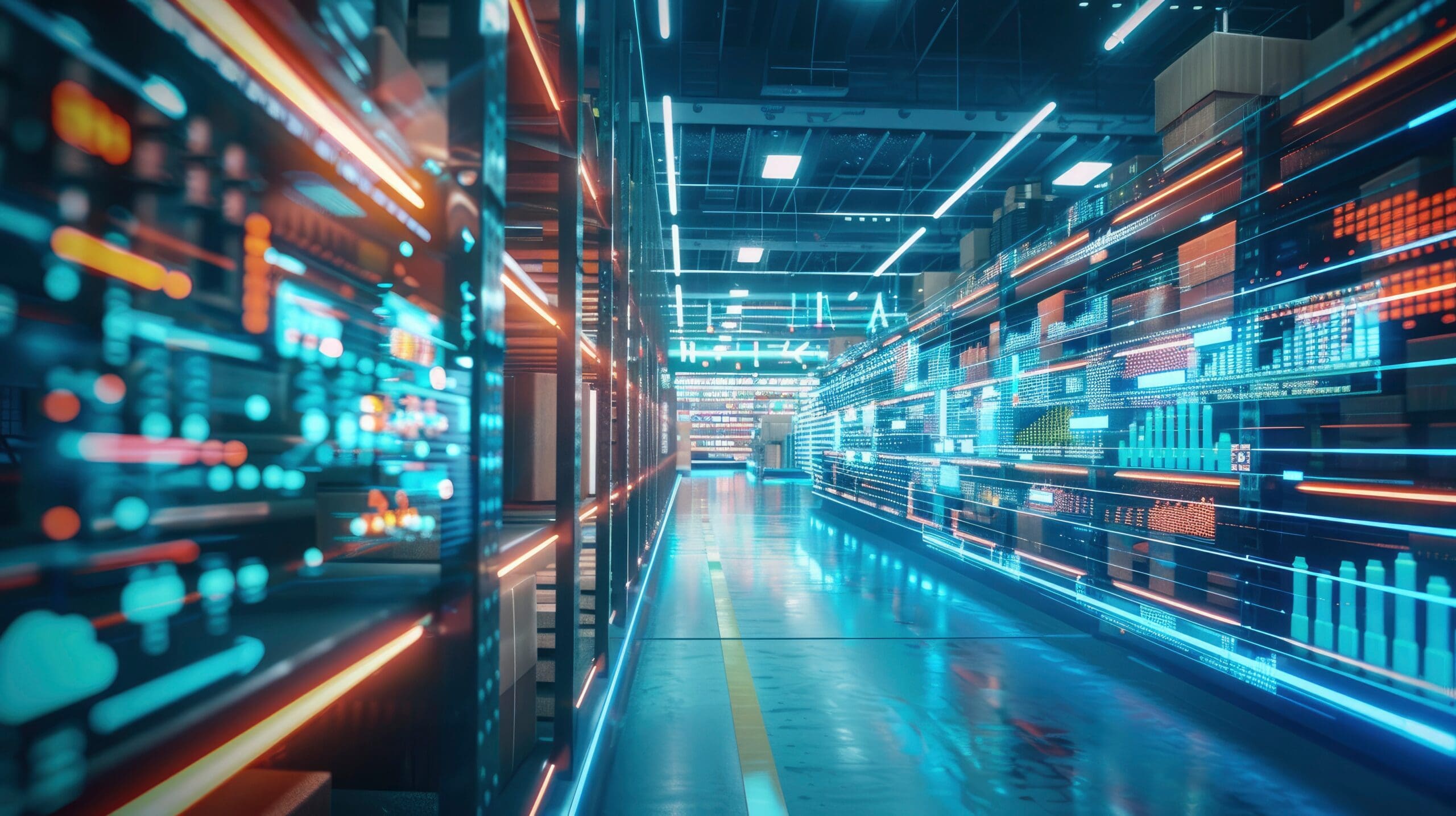
Within manufacturing, business intelligence refers to the systemic use of data analysis tools to inform manufacturing decisions. Data from widely varying sources is collected to obtain actionable insights for improving operational efficiency, productivity, and profitability. FactoryTalk DataMosaix by Rockwell Automation is one such scaleable tool furthering a facility’s progression toward digitalization.
Author contributor: E Tech Group Director, Manufacturing Intelligence & Advanced Services Pradeep Paul
A vast majority of manufacturers list smart manufacturing (data-driven decision making) as important to their future success. Process data is collected within every PLC, HMI, IoT sensor, and meter. This data contains valuable information related to machine performance, environmental conditions, and overall production rates. However, despite its availability, most manufacturers struggle to effectively analyze, and therefore utilize this data in a meaningful way. This lack of data use results in today’s facilities being generally data-rich but paradoxically information-poor. The ability to make data-driven decisions and fully leverage smart manufacturing technologies is therefore hindered.
E Tech Group collaborates with manufacturers to access these valuable insights stemming from operations data for problem-solving and process optimization. This is the field of data analytics for manufacturing-specific business intelligence – manufacturing intelligence.
Analytics can give insights within a hierarchy of four broad categories: descriptive, diagnostic, predictive, and prescriptive information. Each of these categories is expanded upon in Table 1: Types of Analytics. Today, most manufacturing sites are pursuing the first level of analytics, obtaining descriptive insights for systems ranging in size and scale from a singular machine to a particular production line to the enterprise as a whole. At this first level, analytics provide observational insights that may not otherwise be clear. As an example, analytics can answer machine-level questions such as, “How long did the machine run without a fault?” or “What is the most commonly occurring fault?”
However, as can be seen within Table 1, the potential for analytics to answer questions, solve problems and optimize processes goes much further. With the right data, analytics can offer diagnostics-level insights, providing answers for the reasons why a particular observation is occurring. Considering the machine level again, diagnostics analytics answers questions such as, “Why is the fault happening?”
Reviewing the right side of Table 1, analytics can provide even more complex insights for predicting behavior such as knowing the conditions that will produce the particular fault. It can also be used to formulate prescriptive solutions – knowing how to avoid the fault.
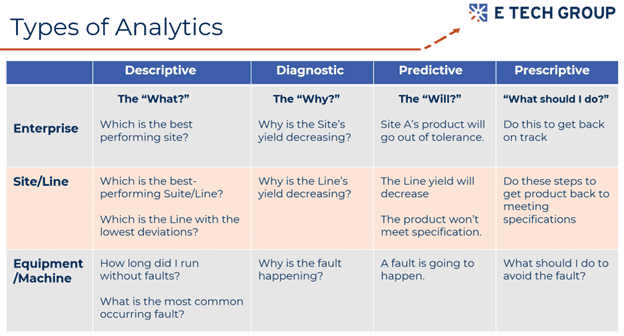
The History of Business Intelligence
In the 2010 timeframe, virtualization technologies gained popularity by allowing for the creation of multiple simulated environments from a single, physical hardware system. This technology led to widespread adoption of virtual machines (VM) – a software-based emulation of a physical computer. Virtualization technology allows for several instances of VMs to run simultaneously and independently on the same physical machine. In essence, VMs maximize the use of physical computer hardware resources while minimizing their costs.
The concept of virtualization laid the foundation for the cloud computing model offering on-demand computing resources over the internet. As more data became available within manufacturing sites, engineers found ways of analyzing it to obtain operational insight. As this process matured, in the 2010-2020 timeframe, the use of data sciences and analytics truly took hold within manufacturing. Analysis tools became increasingly user-friendly and accessible. They no longer required an individual to understand the code behind them to effectively make use of their insights. Rather, an individual only needed to know the right model to select for a particular dataset to reap its benefits.
Software as a service, or SaaS-based products which include those used for manufacturing intelligence evolved from this foundation. Manufacturing intelligence software solutions include cloud-based comprehensive analytics with site-level connectors facilitating data movement from the site to the cloud portion of the application.
An important first step towards using data analytics for decision-making purposes is to identify the specific outcomes a manufacturer aspires to achieve. These outcomes vary widely, including process optimization, root cause analysis of system failures, or making predictions. Focusing on the desired outcomes informs the selection, and therefore the source, of required datasets.
The primary challenge for implementing manufacturing intelligence is that the needed data often resides in very different systems with limited or no streamlined generalized access – commonly termed “data silos.” As an example, data from quality testing may reside in a manufacturing engineer’s spreadsheet to which general operators have no access. Furthermore, the data may lack important context needed for the full use of analytics. Specifically, temperature data may be present but the more precise information describing the location where the measurement was taken may be missing.
E Tech Group’s strategy for implementing a manufacturing intelligence system is as follows:
- Determine the type of decisions that the data analytics system will inform. This clarification will establish how and by whom the data is used to make decisions.
- Locate the required datasets and consolidate into a singular platform.
- Address any further context needed for the data to be useful.
- Select the appropriate platform(s) to analyze the data.
Data Analytics Platforms
While E Tech Group partners with several vendors offering unique SaaS-based analytics solutions, this article focuses on FactoryTalk DataMosaix by Rockwell Automation.
FactoryTalk DataMosaix by Rockwell Automation
FactoryTalk DataMosaix is a software solution by Rockwell Automation that centralizes and organizes data from various sources across a manufacturing operation into a unified namespace (UNS), enabling better data visibility and analysis to improve decision-making and operational efficiency.
Situated between the IT and OT networks (within the iDMZ), DataMosaix can access data originating from devices residing deep within the OT network and also connect to cloud-based portions of the application for complex analysis purposes. While the concept of a UNS presents many practical issues in regard to operations control, it offers significant benefits for reporting and analysis by consolidating data from various sources into a centralized, accessible location. This centralization makes data more usable across different applications improving overall data management.
Rockwell Automation has designed the tool to be a nearly seamless integration for Rockwell-based facilities. DataMosaix uses edge connectors as interface points that collect data from PLCs, HMIs, sensors, and other devices operating on the plant floor. The edge connectors standardize the data and transmit it to the Edge Manager within the DataMosaix system.
Situated within the iDMZ of a facility’s network, the Edge Manager processes the data received from edge connectors and applies local decision-making algorithms to enhance operational efficiency directly at the source. For example, if data coming from an edge connector indicates that a temperature exceeds its operating range, the edge manager can make real-time decisions to adjust the cooling system accordingly, or alert staff to do so. It reserves data transmission to the cloud for more intricate and complex analysis of long-term patterns and trends. The result is a robust data-management system that provides remarkable visibility and analysis of plant operations.
Application-specific SaaS solutions can be purchased via subscription to visualize and interpret specific types of data for further insights. Some examples include:
- Energy Manager: Using utility meters (for electricity, gas, water) installed throughout the target system, Energy Manager provides insight into resource use per process or per machine. Potential outcomes include discernment of the most energy-expensive products to manufacture and determination of the most energy-expensive equipment to operate.
A Note on Cloud-Based Platforms: Security and Convenience
Nearly all manufacturing intelligence solutions are cloud-based SaaS applications. While it is common for manufacturers to be concerned about potential security issues stemming from sending their data to a cloud-based application, it is E Tech Group’s opinion and experience that cloud-based systems are more secure than manufacturing sites. To clarify, it is typically much easier for a cyber-attacker to gain access to a manufacturing facility network than a cloud-based system.
Cloud Security
Cloud applications typically use fully encrypted SSL (Secure Sockets Layer) and TLS (Transport Layer Security) protocols for communications to ensure data confidentiality and integrity throughout transmission.
In addition, cloud service providers follow best practices for security and adhere to industry-specific standards including ISO 27001 and SOC2 which are both important for protecting sensitive manufacturing data. For the purposes of analytics, the data sent to cloud-based applications tends to be anonymized, making it difficult or impossible for a bad actor to use.
Cloud Convenience
By eliminating the need to manage complex IT infrastructure, cloud-based solutions offer significant convenience. Cloud services reduce the burden on IT support by eliminating the need to handle servers, network infrastructure, backups, licenses, and security measures that come with a local system. Additionally, concerns about downtime risks due to power loss and system reliability are mitigated, as the cloud provider is responsible for them.
What is a UNS?
In Manufacturing OT systems, Unified Namespace (UNS) is a conceptual model of a centralized data platform that aggregates and organizes data from numerous sources across the plant floor, enabling seamless data access and interaction among different systems, devices, and applications. This approach allows for interoperability and facilitates more efficient data management and decision-making within a manufacturing environment.
- PlantPAx Analytics provides a centralized location for alarm review. The application is designed to aggregate alarms from different sites/manufacturing lines and provide analytics based on ISA18.2 Alarm Management Standards. This tool facilitates site alarm rationalization efforts so that every system alarm is meaningful and requires operator action. Alarm rationalization eliminates nuisance alarms that typically clutter up the entire alarm system resulting in operator alarm apathy and masking of critical alarms.
E Tech Group is currently engaging with Rockwell Automation to expand this tool for use with other non-Rockwell systems.
- Batch Analytics analyzes data from batch processing to identify optimal production conditions – the golden batch. The software compares current production data to the parameters associated with a golden batch to suggest real-time process adjustments to minimize batch variability, improving overall quality and consistency.
With the full maturity of SaaS infrastructure, the field of manufacturing intelligence stands poised to help manufacturers gather, analyze, and act upon the information locked within disparate data sources from vastly different areas of their operation. This information is invaluable to obtaining both a deep understanding of their current operation, and perhaps more importantly, actionable ways to optimize it. Insights gleaned from manufacturing intelligence tools are a key driver for future competitiveness, innovation, and business success.
This article originally appeared on AutomationWorld.com
Contact Us For Column
Reach out to us here and someone will respond within 24 hours.
"*" indicates required fields